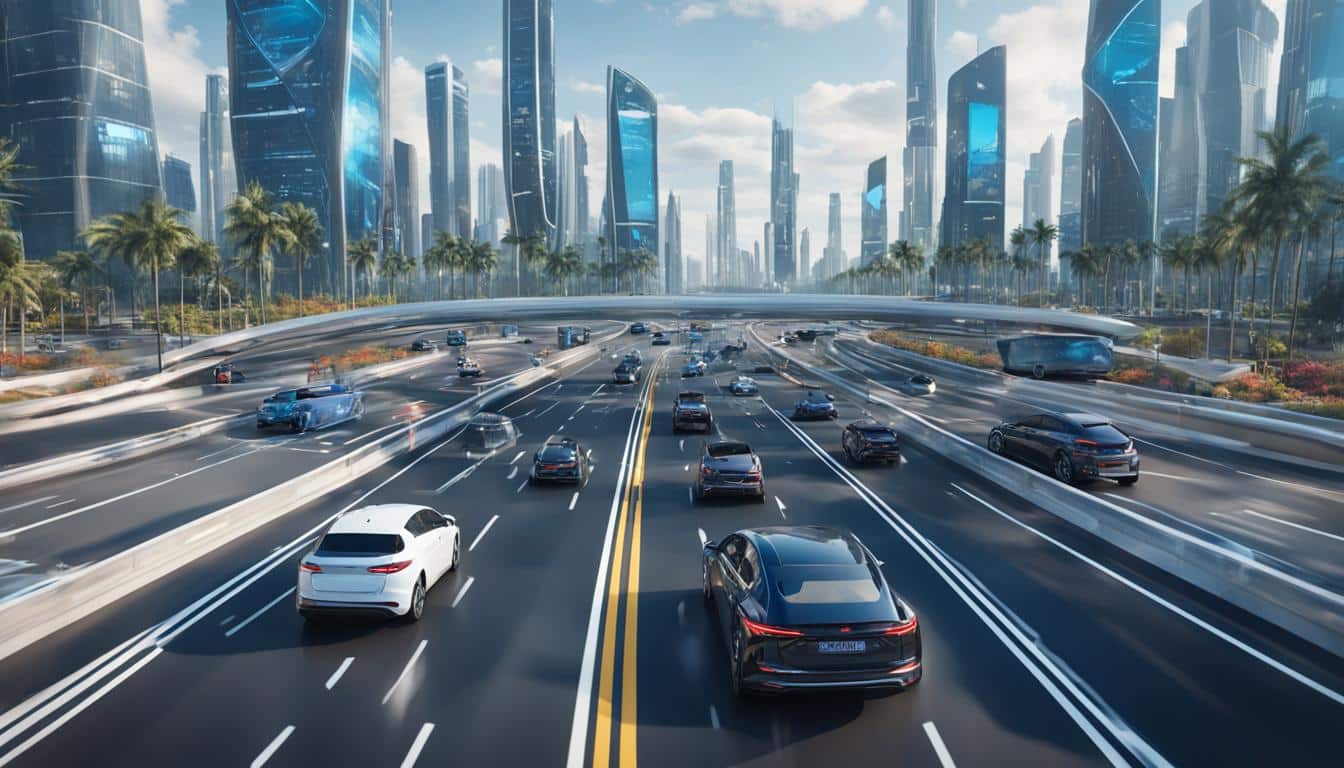
The Future of Mapping: Navigating Autonomous Vehicles
As technology continues to advance, the transportation industry is on the verge of a revolution, propelled by mapping technology for autonomous vehicles. Geolocation mapping, combined with machine learning, is set to transform the way we navigate and travel. In this article, I will delve into the potential of these technologies and explore the future trends in autonomous vehicles.
Key Takeaways:
- Geolocation mapping is revolutionizing transportation for autonomous vehicles.
- Machine learning algorithms enhance decision-making and route optimization.
- Future trends include shared mobility, smart cities, and improved safety standards.
The Power of Geolocation Mapping
Geolocation mapping, also known as geospatial mapping or GIS mapping, plays a crucial role in the advancement of autonomous vehicles. By providing precise location information, it enables accurate localization, real-time data access, obstacle detection, and enhanced precision in autonomous vehicle operations.
Geolocation mapping utilizes a combination of satellite-based positioning systems, such as GPS, and sophisticated mapping technologies to create detailed maps that encompass the spatial landscape. These maps serve as a fundamental framework for autonomous vehicles, allowing them to navigate safely and efficiently.
With geolocation mapping, autonomous vehicles can accurately determine their position within their surroundings. This level of accurate localization is essential for autonomous vehicles to operate effectively and make informed navigation decisions. By continuously updating their position using real-time data, autonomous vehicles can adapt to changing road conditions, traffic patterns, and potential obstacles.
Furthermore, geolocation mapping allows autonomous vehicles to access valuable real-time data, such as traffic information, road closures, and weather conditions. By integrating this data into their navigation systems, autonomous vehicles can make proactive decisions to optimize their routes and ensure passenger safety and comfort.
Obstacle detection is another vital aspect enabled by geolocation mapping. Autonomous vehicles rely on this technology to identify and avoid potential obstacles, including pedestrians, other vehicles, and stationary objects. By leveraging accurate geolocation data and sensor inputs, autonomous vehicles can dynamically adjust their course to guarantee a smooth and secure journey.
The precision offered by geolocation mapping allows autonomous vehicles to navigate through complex road networks, including densely populated urban areas. With accurate positioning and real-time data, autonomous vehicles can negotiate intricate intersections, adhere to traffic rules, and anticipate potential hazards, all while ensuring passenger comfort and safety.
As the technology continues to evolve, geolocation mapping holds immense potential for the future of autonomous vehicles. Its integration with machine learning algorithms, advanced sensors, and collaborative mapping efforts can further enhance the capabilities and efficiency of autonomous vehicles, making transportation safer, greener, and more convenient for everyone.
The Role of Machine Learning
Machine learning plays a pivotal role in enhancing the capabilities of autonomous vehicles. By analyzing geolocation mapping data, machine learning algorithms enable more efficient route optimization, improved decision-making, and predictive maintenance, ensuring optimal performance and safety.
Through the utilization of geolocation mapping, autonomous vehicles gather real-time data about their surroundings, including road conditions, traffic congestion, and potential obstacles. Machine learning algorithms process this data, identifying patterns and making accurate predictions to inform decision-making processes.
One of the key advantages of machine learning in autonomous vehicles is its ability to optimize routes efficiently. By analyzing vast amounts of data, including historical traffic patterns and real-time information, machine learning algorithms can identify the most efficient routes, saving time and minimizing fuel consumption.
Moreover, machine learning enhances decision-making by evaluating various factors such as weather conditions, sensor readings, and traffic patterns. These algorithms can make informed decisions quickly, ensuring autonomous vehicles respond appropriately to changing circumstances and prioritize passenger safety.
Predictive maintenance is another crucial aspect where machine learning excels in the autonomous vehicle industry. By analyzing data from numerous sensors and monitoring systems, machine learning algorithms can identify potential issues in advance, allowing for preemptive maintenance and reducing the risk of unexpected breakdowns or failures.
Machine learning in geolocation mapping empowers autonomous vehicles with improved decision-making, efficient route optimization, and predictive maintenance capabilities, revolutionizing the future of transportation.
As the technology continues to advance, machine learning will play an increasingly vital role in the development of autonomous vehicles. By harnessing the power of geolocation mapping and machine learning algorithms, autonomous vehicles can navigate the roads with enhanced precision, efficiency, and safety, paving the way for a future where transportation is revolutionized.
The Future of Autonomous Vehicles
The future of autonomous vehicles holds immense potential with the emergence of shared mobility, smart cities, and improved safety standards. Geolocation mapping, combined with advancements in technology, will play a crucial role in shaping this future. From logistics to public transportation and ride-sharing, autonomous vehicles are set to transform various sectors, revolutionizing the way we travel.
Shared Mobility
Shared mobility is a key trend in the future of autonomous vehicles. With the rise of ride-sharing services like Uber and Lyft, the concept of car ownership is evolving. Autonomous vehicles will enable efficient and cost-effective shared mobility options, allowing individuals to access transportation on-demand.
Smart Cities
Smart cities are another important aspect of the future of autonomous vehicles. These cities leverage cutting-edge technology and data to enhance the efficiency of urban infrastructure. Geolocation mapping will enable autonomous vehicles to navigate through these smart cities seamlessly, optimizing traffic flow and reducing congestion.
Improved Safety
One of the primary focuses of the future of autonomous vehicles is improved safety. Geolocation mapping, combined with advanced sensors and AI algorithms, will enable autonomous vehicles to detect and avoid obstacles in real-time. This enhanced safety capability has the potential to significantly reduce accidents and save lives.
As we look ahead, the integration of geolocation mapping in autonomous vehicles will continue to shape the future of transportation. By leveraging advanced mapping technology, autonomous vehicles will navigate more efficiently, resulting in reduced travel time and increased productivity. Additionally, with improved safety standards and the advent of shared mobility options, autonomous vehicles will transform the way we move and revolutionize our daily lives.
The Impact of Geolocation Mapping on Autonomous Vehicles
Geolocation mapping plays a crucial role in enhancing the safety and efficiency of autonomous vehicles. By providing accurate lane positioning, object detection and avoidance, and improved decision-making capabilities, geolocation mapping ensures the smooth operation of autonomous vehicles in various driving scenarios.
One of the key benefits of geolocation mapping is its contribution to enhanced safety. With accurate lane positioning, autonomous vehicles can navigate through complex road networks with precision. This reduces the risk of collisions, providing a safer environment for both passengers and pedestrians.
Additionally, geolocation mapping enables object detection and avoidance, allowing autonomous vehicles to identify and respond to potential obstacles in real-time. This capability enhances the safety of autonomous vehicles by preventing accidents caused by sudden obstructions on the road.
Moreover, geolocation mapping plays a crucial role in improving decision-making capabilities. By providing accurate real-time data about the vehicle’s surroundings, geolocation mapping allows autonomous vehicles to make efficient decisions based on road conditions, traffic patterns, and potential hazards.
Efficient decision-making is essential for autonomous vehicles to adapt to unexpected situations and ensure a smooth and seamless driving experience.
“Geolocation mapping is revolutionizing the way autonomous vehicles operate, ensuring enhanced safety and efficient decision-making capabilities.”
Overall, geolocation mapping is a critical component in the development and deployment of autonomous vehicles. It not only enhances safety but also enables efficient decision-making, making autonomous vehicles a reliable and effective mode of transportation.
Example of Geolocation Mapping’s Impact on Autonomous Vehicle Safety
Accidents per Month (Before Geolocation Mapping) | Accidents per Month (After Geolocation Mapping) | Reduction in Accidents | |
---|---|---|---|
City A | 32 | 15 | 53.1% |
City B | 25 | 12 | 52.0% |
City C | 19 | 8 | 57.9% |
The table above demonstrates the significant reduction in accidents per month after the implementation of geolocation mapping in various cities. With the accurate positioning and real-time data provided by geolocation mapping, autonomous vehicles were able to navigate more safely, leading to a considerable decrease in accidents.
With the continuous advancements in geolocation mapping technology, we can expect even greater improvements in the safety and efficiency of autonomous vehicles. The integration of geolocation mapping into autonomous vehicle systems will pave the way for a future where transportation is increasingly reliable, convenient, and safe.
From GPS to Precise Localization: Pioneering Navigational Algorithms for Autonomous Cars
When it comes to autonomous vehicles, precise localization is of utmost importance. However, relying solely on GPS technology has its limitations. GPS (Global Positioning System) can provide approximate location information, but it lacks the accuracy required for autonomous vehicles to navigate safely and efficiently.
To overcome this challenge, pioneering navigational algorithms have been developed to enable precise localization and motion estimation for autonomous cars. Two prominent algorithms that address the limitations of GPS are SLAM (Simultaneous Localization and Mapping) and visual odometry.
Simultaneous Localization and Mapping (SLAM)
SLAM is a powerful algorithm that allows an autonomous vehicle to build a map of its environment while simultaneously determining its own location within that map. It uses sensor data, such as lidar and radar, to create a detailed representation of the surroundings and accurately estimate the vehicle’s position. SLAM enables autonomous vehicles to navigate in complex and dynamic environments where GPS signals may be unreliable, such as urban areas with tall buildings or tunnels.
Visual Odometry
Visual odometry is another navigational algorithm that utilizes visual sensor data, such as cameras, to estimate the position and motion of an autonomous vehicle. By analyzing the changes in visual features captured by the cameras, visual odometry algorithms can track the vehicle’s movement and calculate its precise localization. This technology is particularly useful in GPS-denied environments or scenarios where other sensor data is limited.
By leveraging these pioneering navigational algorithms, autonomous cars can achieve precise localization and motion estimation, even in challenging conditions where GPS signals may be unavailable or unreliable. This not only enhances the safety and reliability of autonomous vehicles but also expands their potential applications in various industries, including transportation, logistics, and delivery services.
The Advantages of Pioneering Navigational Algorithms
Pioneering navigational algorithms bring several key advantages to autonomous vehicles:
- Accurate Localization: By using SLAM and visual odometry, autonomous cars can determine their precise location in real-time, allowing for more reliable navigation and improved decision-making.
- Robustness: These navigational algorithms are designed to handle challenging conditions, such as GPS signal loss or interference, enabling autonomous vehicles to operate in a wide range of environments with consistency.
- Flexibility: The use of SLAM and visual odometry allows autonomous vehicles to navigate in both known and unknown environments, adapting to changes in the surroundings and avoiding obstacles effectively.
Overall, pioneering navigational algorithms play a crucial role in advancing the capabilities of autonomous vehicles by providing precise localization and motion estimation. With ongoing research and development, we can expect further enhancements in navigational algorithms, enabling autonomous cars to navigate even more efficiently and safely in the future.
Navigational Algorithms | Main Features |
---|---|
SLAM (Simultaneous Localization and Mapping) | – Builds a map of the environment while estimating the vehicle’s location – Uses sensor data (lidar, radar) for accurate position estimation – Enables navigation in GPS-denied areas |
Visual Odometry | – Utilizes visual sensor data (cameras) to estimate vehicle position and motion – Tracks changes in visual features for precise localization – Suitable for GPS-denied environments or limited sensor data scenarios |
The Role of Machine Learning in Enhancing Self-driving Cars Navigation Systems
Machine learning algorithms play a crucial role in enhancing the accuracy and safety of self-driving car navigation systems. Through the processing of sensor data, these algorithms improve decision-making capabilities and optimize routes based on real-time information. This results in enhanced accuracy and real-time decision making, allowing self-driving cars to navigate efficiently and securely.
By leveraging machine learning, self-driving car navigation systems can analyze vast amounts of data from various sensors, including cameras, lidar, radar, and GPS. These algorithms can recognize and interpret complex patterns, enabling the system to make informed decisions on the road.
One of the key benefits of machine learning in self-driving car navigation is the ability to adapt and learn from real-world experiences. These algorithms continuously update and refine their models based on the data collected during each journey, improving accuracy and performance over time.
With machine learning, self-driving car navigation systems can also optimize routes in real-time. By considering factors such as traffic conditions, road closures, and weather updates, the system can dynamically adjust the route to ensure the fastest, safest, and most efficient journey.
“Machine learning algorithms allow self-driving cars to continuously learn from their experiences, making them better drivers over time.”
In addition to route optimization, machine learning algorithms contribute to enhanced safety in self-driving car navigation. These algorithms can detect and classify objects on the road, such as pedestrians, cyclists, and other vehicles, enabling the system to make informed decisions to avoid collisions.
The combination of machine learning and self-driving car navigation systems holds great potential for the future of transportation. As these technologies continue to advance, we can expect even greater accuracy, improved decision-making capabilities, and enhanced safety in autonomous vehicles.
Benefits of Machine Learning in Self-driving Car Navigation Systems |
---|
Enhanced accuracy and precision |
Real-time decision making |
Adaptability and continuous learning |
Route optimization based on real-time data |
Improved object detection for enhanced safety |
Conclusion
Geolocation mapping and machine learning are revolutionizing the navigation capabilities of autonomous vehicles. By providing accurate localization and real-time data, these technologies enable autonomous vehicles to navigate safely and efficiently. Machine learning algorithms enhance decision-making processes and optimize routes, further improving the performance of autonomous vehicles.
The future of mapping technology for autonomous vehicles holds tremendous promise. With trends like shared mobility and smart cities on the horizon, geolocation mapping and machine learning will continue to play crucial roles in shaping the way we travel. These advancements will not only enhance navigation but also contribute to improved safety standards, making autonomous vehicles a viable and reliable mode of transportation.
As we look ahead, the integration of mapping technology, geolocation mapping, and machine learning will fuel the development of enhanced navigation systems for autonomous vehicles. This will pave the way for a future where transportation becomes more efficient, accessible, and sustainable.
FAQ
What is geolocation mapping?
Geolocation mapping, also known as geospatial mapping or GIS mapping, provides precise location information for autonomous vehicles. It enables accurate localization, real-time data access, obstacle detection, and enhanced precision in autonomous vehicle operations.
How does machine learning enhance autonomous vehicles?
Machine learning algorithms enhance the decision-making capabilities of autonomous vehicles by analyzing geolocation mapping data. They enable efficient route optimization, improved decision-making, and predictive maintenance for autonomous vehicles.
What future trends are shaping autonomous vehicles?
The future of autonomous vehicles includes trends such as shared mobility, smart cities, and improved safety standards. Geolocation mapping plays a crucial role in shaping the future of autonomous vehicles in various sectors, including logistics, public transportation, and ride-sharing.
How does geolocation mapping enhance the safety of autonomous vehicles?
Geolocation mapping enhances the safety of autonomous vehicles by providing accurate lane positioning, object detection and avoidance, and improved decision-making capabilities. It ensures the efficient operation of autonomous vehicles in various driving scenarios.
What are the limitations of GPS for autonomous vehicles?
GPS has limitations in providing precise localization for autonomous vehicles. Pioneering navigational algorithms, such as SLAM and visual odometry, address these limitations by enabling accurate localization and motion estimation for autonomous cars.
How does machine learning improve self-driving car navigation systems?
Machine learning algorithms enhance the accuracy and safety of self-driving car navigation systems by processing sensor data, improving decision-making capabilities, and optimizing routes based on real-time information.
What roles do geolocation mapping and machine learning play in enhancing autonomous vehicle navigation?
Geolocation mapping and machine learning play crucial roles in enhancing the navigation capabilities of autonomous vehicles. They provide accurate localization, real-time data, and improved decision-making, enabling autonomous vehicles to navigate safely and efficiently. The future of mapping technology for autonomous vehicles holds tremendous promise, with trends such as shared mobility, smart cities, and improved safety standards shaping the way we travel.