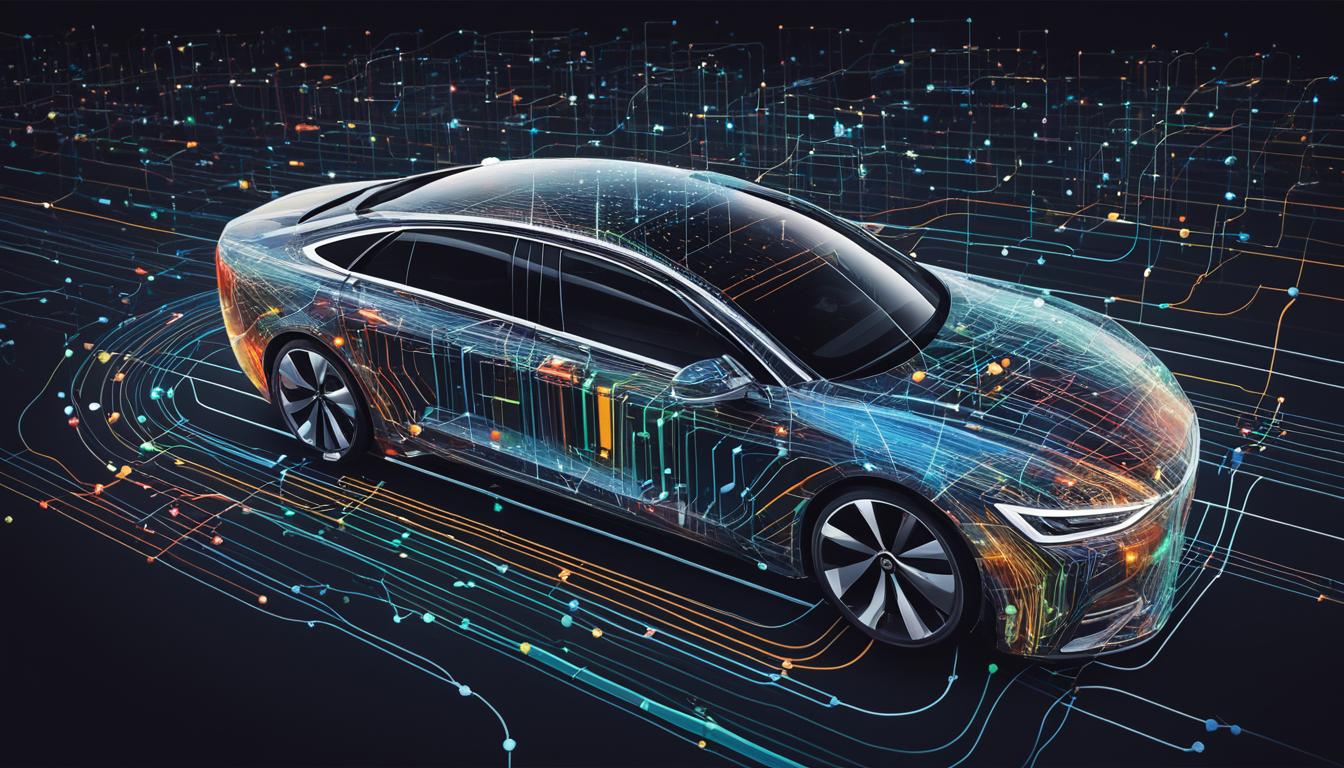
Big Data’s Crucial Role in Self-Driving Car Development
Self-driving car technology has made remarkable progress in recent years, with major tech companies and car manufacturers collaborating to develop advanced autonomous driving functions. As we move closer to the public release of fully automated vehicles, it becomes evident that big data has played a vital role in driving this transformation. By providing the programming data necessary for self-driving cars to become safer and more efficient, big data is revolutionizing the automotive industry.
Key Takeaways:
- Big data is playing a crucial role in the development of self-driving cars.
- The evolution of self-driving cars can be traced back to the invention of cruise control in 1950.
- Self-driving cars rely on data received from GPS, radar, sensors, and cameras to make safe-driving decisions.
- Data-driven sensory perception enables self-driving cars to accurately detect and interpret their surroundings.
- Machine learning algorithms are essential in training self-driving cars to operate in complex traffic scenarios.
History of Self-Driving Cars
The evolution of self-driving cars traces back to the invention of cruise control in 1950. This revolutionary feature allowed drivers to set a constant speed for their vehicles, providing a precursor to the automation seen in modern self-driving cars. Building upon this foundation, numerous automated functions have been introduced over the years to improve safety and reduce human error.
One such advancement is proximity warnings, which use sensors to alert drivers to potential dangers on the road. By detecting objects or vehicles in close proximity, these systems help prevent accidents caused by inattentiveness or distracted driving.
Additionally, innovations like adaptive cruise control automatically maintain a safe distance from the vehicle ahead, adjusting the speed accordingly. This technology utilizes sensors and algorithms to monitor the surrounding traffic, promoting smoother and safer driving experiences.
Despite these remarkable advancements, human error remains a significant contributor to accidents on the road. Studies show that approximately 90% of all car accidents are caused by human mistakes, such as distracted driving, speeding, or impaired judgement. The limitations of human drivers have highlighted the need for fully autonomous vehicles that can eliminate the potential for human error completely.
“Human error accounts for approximately 90% of all car accidents.”
Image:
Big Data’s Role in Autonomous Vehicle Development
Big data plays a crucial role in the development of autonomous vehicles. These vehicles rely on data received from GPS, radar, sensors, and cameras to make safe-driving decisions. Car manufacturers use big data to program self-driving cars to handle various scenarios they may encounter on the road. As the price of advanced radars decreases, self-driving cars will become more accessible to the public, leading to increased road safety worldwide.
The Importance of GPS Data
GPS technology is a fundamental component of autonomous vehicle development. It provides precise location information that enables self-driving cars to navigate their surroundings accurately. GPS data, combined with other sensory inputs, allows autonomous vehicles to plan routes, anticipate traffic conditions, and make efficient decisions in real-time.
The Role of Radar and Sensors
Radar technology and sensors are critical for autonomous vehicles to detect and avoid potential obstacles on the road. Radar systems use radio waves to measure the distance and speed of surrounding objects, providing essential data for self-driving cars to calculate safe distances and adjust their driving behavior accordingly. Sensors, on the other hand, capture information about the vehicle’s surroundings, such as the presence of pedestrians, cyclists, or other vehicles.
“Big data enables car manufacturers to program self-driving cars to handle various scenarios they may encounter on the road.”
Data-Driven Programming
Programming self-driving cars involves developing complex algorithms that interpret the vast amounts of data collected from GPS, radar, sensors, and other sources. By analyzing and processing this data, car manufacturers can create robust programming models that allow autonomous vehicles to make accurate and safe driving decisions in real-time. The continuous collection and analysis of big data contribute to the improvement and refinement of these programming models, enhancing the overall performance and reliability of self-driving cars.
The Role of Big Data in Autonomous Vehicle Development
Data Source | Role |
---|---|
GPS | Provides precise location data for navigation and route planning. |
Radar | Enables detection and avoidance of obstacles on the road. |
Sensors | Capture information about the vehicle’s surroundings, including the presence of pedestrians, cyclists, and other vehicles. |
As the table above illustrates, big data from GPS, radar, and sensors contributes to different aspects of autonomous vehicle development, ensuring accurate and safe driving decisions.
As the image above visually represents, the integration of big data and advanced technologies in autonomous vehicle development is paving the way for safer, more efficient, and reliable transportation systems of the future.
Data-Driven Sensory Perception
In the development of self-driving cars, one of the crucial challenges is equipping these vehicles with the ability to perceive and interpret their surroundings accurately. This is where data-driven sensory perception plays a vital role, harnessing the power of big data to enable self-driving algorithms to process and analyze real-time information from sensors.
Thanks to data-driven sensory perception, self-driving cars can detect and predict the behavior of pedestrians, cyclists, other vehicles, road signs, and traffic signals. By continuously collecting and analyzing data from various sources, these vehicles can make accurate and safe decisions on the road, ensuring the well-being of both passengers and other individuals sharing the streets.
Let’s take a closer look at some of the key elements that rely on data-driven sensory perception in self-driving car development:
- Pedestrians: Self-driving cars use data from sensors to identify pedestrians in their vicinity. Through advanced algorithms, these vehicles can predict their movements and take appropriate actions to avoid accidents.
- Cyclists: Recognizing and understanding the behavior of cyclists is crucial for self-driving cars to navigate safely on the roads. By analyzing sensor data, self-driving algorithms can account for cyclists’ movements and adjust their driving behavior accordingly.
- Road Signs: Data-driven sensory perception allows self-driving cars to interpret and respond to various road signs accurately. By processing information from cameras and sensors, these vehicles can understand speed limits, stop signs, and other important traffic indications.
- Traffic Signals: Understanding traffic signals is essential for safe and efficient driving. Self-driving cars rely on data-driven sensory perception to detect and interpret traffic signals, ensuring compliance with traffic rules and seamless traffic flow.
Data-driven sensory perception in self-driving cars is an ongoing area of research and development. As technology advances and more comprehensive datasets become available, the accuracy and reliability of these systems will continue to improve, bringing us closer to a future where autonomous vehicles dominate the roads.
By leveraging the power of big data and enhancing sensory perception, self-driving cars have the potential to revolutionize transportation, offering safer and more efficient journeys for all.
Machine Learning and Decision-Making
Machine learning plays a pivotal role in training self-driving cars to navigate complex traffic scenarios. These cars rely on advanced algorithms that continuously learn from real-time data and make informed decisions on the road. The training data, derived from big data, enables these algorithms to understand and react to various driving situations.
Self-driving cars collect massive amounts of data during their operations, including sensor readings, GPS coordinates, and video feeds. This data is then processed and analyzed to improve their driving behavior and enhance safety protocols. As self-driving cars encounter new scenarios, the collected data is used to refine their decision-making models.
The continuous learning process allows self-driving cars to become more reliable and proficient over time. By leveraging big data and machine learning, these vehicles are equipped to make informed decisions, prioritize safety, and optimize their driving performance.
Moreover, the integration of machine learning algorithms allows self-driving cars to adapt to changing road conditions and unexpected events. Their decision-making capabilities are designed to identify potential risks, predict and react to the behavior of other vehicles, pedestrians, and road signs.
Self-driving cars rely on machine learning and data collection to improve their ability to navigate complex traffic scenarios and make informed decisions. Through continuous learning and refinement of decision-making models, these vehicles become more reliable and efficient over time.
Real-Time Analysis and Response
Real-time data analysis is crucial for self-driving cars to make split-second decisions and respond to dynamic driving conditions. The continuous processing of data streams collected by onboard sensors enables self-driving cars to adapt their behavior instantaneously. This real-time analysis ensures the safety of passengers and allows self-driving cars to navigate securely on the road.
Self-driving cars rely on a multitude of sensors to collect data from their surroundings in real-time. These sensors include cameras, LiDAR, radar, and ultrasonic sensors, among others. Each sensor captures specific information, such as the position of other vehicles, road conditions, and obstacles, which are then processed and analyzed to inform the self-driving car’s decision-making.
For instance, the self-driving car’s sensor system can detect pedestrians crossing the road and calculate their speed and trajectory. Through real-time analysis, the car can make instantaneous adjustments to its speed and direction to avoid potential collisions. By continuously analyzing data streams, self-driving cars can perceive their environment and respond promptly to changing situations.
Real-time analysis is particularly essential when encountering challenging driving scenarios, such as unexpected obstacles or rapidly changing traffic conditions. By swiftly processing incoming data, self-driving cars can make informed decisions in real-time, enhancing the overall safety and efficiency of autonomous driving.
“The ability of self-driving cars to analyze and respond to data streams in real-time represents a significant advancement in the field of autonomous driving. This capability allows these vehicles to navigate complex road situations, ensuring the safety of passengers and other road users.”
The implementation of real-time analysis in autonomous vehicles has been made possible by the rapid development of computing technologies and sophisticated algorithms. These advancements enable self-driving cars to handle the vast amount of data generated by their sensors in real-time.
Data Stream Processing
Data stream processing is a critical component of real-time analysis in self-driving cars. It involves capturing, filtering, and analyzing data in motion to extract valuable insights and make instant decisions. The collected data streams are processed through powerful onboard computers equipped with advanced algorithms specifically designed for self-driving applications.
By leveraging high-performance computing systems, self-driving cars can analyze data streams in real-time, identifying patterns, detecting anomalies, and predicting future events. This instantaneous analysis allows self-driving cars to react swiftly, maintaining optimal safety and efficiency in various driving scenarios.
Benefits of Real-Time Analysis | |
---|---|
Enhanced safety | Real-time analysis enables self-driving cars to react promptly to potential hazards, reducing the risk of accidents. |
Optimized driving behavior | By continuously processing data streams, self-driving cars can adjust their speed, trajectory, and response to road conditions, enhancing the overall driving experience. |
Adaptive decision-making | Real-time analysis allows self-driving cars to adapt their decisions based on the latest information, improving efficiency and reducing errors. |
Integration with smart infrastructure | Real-time analysis facilitates the integration of self-driving cars with smart traffic systems, enabling efficient coordination and traffic flow optimization. |
Predictive Maintenance and Enhanced Reliability
Big data not only enhances the driving capabilities of self-driving cars but also plays a critical role in improving their reliability and longevity. The continuous monitoring of various systems and components, coupled with data collection on their performance and health, enables self-driving cars to predict potential failures and schedule maintenance before critical issues arise. This proactive approach, known as predictive maintenance, offers several benefits that contribute to the overall enhanced reliability and performance of self-driving cars.
Predictive maintenance reduces the risk of unexpected breakdowns, ensuring that self-driving cars operate smoothly and reliably. By collecting and analyzing real-time data on the condition of components and systems, self-driving cars can detect early signs of wear and tear or potential failures. This enables timely intervention and necessary repairs, preventing costly breakdowns and minimizing the risk of accidents on the road.
Additionally, predictive maintenance optimizes operational efficiency by maximizing the uptime of self-driving cars. By strategically scheduling maintenance based on data-driven predictions, operators can minimize the downtime of their vehicles, ensuring that more cars are available for use and improving overall fleet efficiency.
Furthermore, predictive maintenance helps reduce maintenance costs associated with self-driving cars. By identifying and addressing potential issues before they escalate, operators can avoid costly repairs and replacements. Additionally, scheduled maintenance can be conducted during off-peak hours, optimizing resources and reducing operational expenses. This cost-saving approach allows operators to allocate their budgets effectively and invest in the continuous improvement and development of self-driving car technology.
Overall, predictive maintenance enabled by big data monitoring and analysis enhances the reliability and longevity of self-driving cars. By proactively addressing potential maintenance issues and optimizing operational efficiency, self-driving cars can offer a safer, more reliable mode of transportation for the future.
Conclusion
Big data is revolutionizing the development and advancement of self-driving car technology. Through the provision of programming data and real-time analysis, big data plays a crucial role in making autonomous vehicles safer, more efficient, and more reliable. As the automotive industry continues to harness the power of big data, the future of transportation is set to be transformed.
By utilizing big data, self-driving cars are equipped with the necessary information to navigate and make decisions in real-time. The extensive data collected from GPS, radar, sensors, and cameras empowers these vehicles to adapt to various scenarios on the road, enhancing safety for passengers and pedestrians alike.
As we move forward, autonomous vehicles have the potential to reshape transportation as we know it. With the continued integration of big data, self-driving cars offer a future that is not only safer, but also more convenient and efficient. Imagine a world where traffic congestion is reduced, accidents become a rarity, and travel time is optimized. This is the future that big data and self-driving car development can bring.
FAQ
What is the role of big data in self-driving car development?
Big data plays a crucial role in the development of autonomous vehicles. It provides the programming data needed for self-driving cars to make safe-driving decisions and handle various scenarios they may encounter on the road.
How do self-driving cars perceive and interpret their surroundings?
Self-driving cars rely on big data to process and analyze real-time information from sensors. This allows them to detect and predict the behavior of pedestrians, cyclists, other vehicles, road signs, and traffic signals, ensuring accurate and safe decision-making.
How do machine learning algorithms contribute to self-driving car development?
Machine learning algorithms are essential for training self-driving cars to operate in complex traffic scenarios. Big data provides the necessary raw materials for training these algorithms, allowing self-driving cars to continuously learn and improve their driving behavior and safety protocols.
How do self-driving cars make split-second decisions?
Self-driving cars rely on real-time data analysis to make split-second decisions and respond to dynamic driving conditions. The data collected by onboard sensors is continuously processed, allowing the car’s behavior to adapt instantaneously and ensure the safety of passengers.
How does big data enhance the reliability and longevity of self-driving cars?
Big data enables self-driving cars to continuously monitor various systems and components, collecting data on their performance and health. With this information, self-driving cars can predict potential failures and schedule maintenance before critical issues arise, reducing unexpected breakdowns and optimizing operational efficiency.
What is the future of self-driving car development?
With the help of big data, self-driving car development is expected to reshape the future of transportation, offering a safer, more efficient, and more convenient mode of transportation. Autonomous vehicles are set to become more accessible to the public, leading to increased road safety worldwide.